首页 >
行业动态 > 【AI与MOF材料】机器学习辅助下的丙烷选择性金属有机框架的发现
【AI与MOF材料】机器学习辅助下的丙烷选择性金属有机框架的发现
摘要:
暨南大学陆伟刚教授/李丹教授团队报道了一个机器学习辅助的高通量计算筛选策略,用于从CoRE MOF数据库中筛选丙烷选择性金属有机框架材料。应用了四种机器学习算法,随机森林算法显示出最高的预测准确度。实验验证了筛选出的JNU-90材料,确认了它优异的丙烷/丙烯选择性和分离性能。考虑到JNU-90也具有良好的水解稳定性,它展示了在能效分离丙烷/丙烯方面的巨大应用潜力。
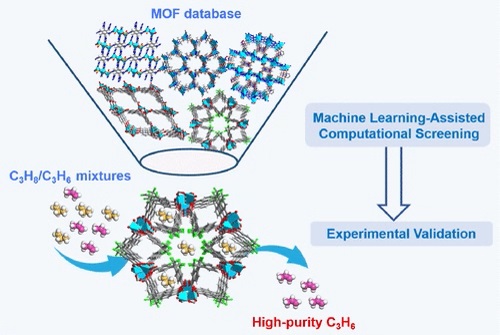
研究背景:
1)丙烯是重要的石化原料,目前工业上主要通过热解和催化裂化生产,但会产生丙烷作为必不可少的副产物。获得聚合级丙烯需要复杂的低温精馏,耗能大。
2)金属有机框架材料被认为是有前景的替代技术,但发展选择性好的丙烷金属有机框架仍然是一个巨大挑战。
3)高通量计算可以快速计算大量框架材料的性能,但计算量巨大。机器学习可以在部分数据的基础上进行训练,然后快速准确地预测全库材料,可以极大地提高效率。
本文通过机器学习辅助的高通量计算筛选,目标是发现丙烷选择性较高的金属有机框架,以期望通过实验验证这种方法的可行性,为开发这类材料提供指导。
实验部分:
1)训练和测试了四种不同的机器学习算法的预测性能,发现随机森林算法预测丙烷/丙烯选择性的相关系数最高,达到0.83。
2)分析了结构和能量描述子与选择性的相关性,发现亨利系数比和差示差热是最重要的两个因素。
3)通过结构和能量筛选,识别出三种预测选择性较高的金属有机框架(PUQXUV、LUDKUS和ZARZAV)。
4)考虑到水解稳定性,选择合成和表征了PUQXUV(JNU-90)。单晶衍射确定了其晶体结构,与文献报道一致。
5)测定了JNU-90的气体吸附性能,确认它对丙烷的优先吸附性和高选择性。
实验小结:
1)合成表征确认JNU-90具有文献报道的结构和性质。
2)气体吸附实验结果与计算预测吻合,确认了该方法的有效性。
结论:
1)机器学习辅助高通量计算筛选可以高效准确地识别丙烷选择性金属有机框架。
2)合成的JNU-90材料表现出色,丙烷/丙烯选择性达到2.7,优于文献报道。
3)该策略可以指导开发更多烃类分离金属有机框架材料。
Figure 1. Schematic workflow of the ML-assisted discovery of the top-performing C3H8-selective MOFs from the CoRE MOF database.
Figure 2. Descriptors of the ML. (a) Relative importance of energy descriptors: adsorption heat difference (ΔQst), Henry coefficient ratio (S0), and
structural descriptors: pore limiting diameter (PLD), largest cavity diameter (LCD), global cavity diameter (GCD), accessible surface area (ASA),
void fraction (VF), crystal density (ρ), and pore volume (Vpore) based on RF algorithm. (b) Plot of ΔQst against S0, the red data points are the 52
MOF structures with their adsorption selectivity higher than 2.0.
Figure 3. Structure−property relationships. Relationships between C3H8/C3H6 selectivity and the four structural descriptors: (a) LCD, (b) PLD,
(c) ASA, and (d) Vpore. The color scale from blue to red represents the increase in VF. The data points in the dashed-line rectangles are the MOF
structures with a C3H8/C3H6 selectivity higher than 2.0.
Figure 4. Crystal structure of JNU-90. (a) A rod-shaped Zn-carboxylate secondary building unit of JNU-90. (b) The coordination mode of the
linker. (c) The crystal structure of JNU-90. (d, e) Channels A and B of JNU-90. Cyan, white, gray, red, and green represent the Zn, H, C, O, and F
atoms, respectively.
Figure 5. (a) N2 adsorption−desorption isotherms of JNU-90 at 77 K; the filled and unfilled symbols represent adsorption and desorption,
respectively. The inset shows the pore size distribution of JNU-90 calculated by using the Horvath−Kawazoe (HK) method. (b) Comparison of N2
adsorption isotherms at 77 K and PXRD patterns of the as-synthesized and the water-treated JNU-90 (being soaked in water for 2 months). (c)
Single-component C3H8 and C3H6 adsorption isotherms of JNU-90 at 298 K. (d) IAST selectivity of JNU-90 for a C3H8/C3H6 (1/1) mixture at
298 K. (e) Comparison of IAST selectivity of representative C3H8-selective MOFs for a C3H8/C3H6 (1/1) mixture at 298 K and 1 bar. (f) Qst of
JNU-90 for C3H8 and C3H6 at 298 K.
展望:
1)进一步优化机器学习模型,提高预测准确度。
2)测试JNU-90在实际烃类混合气体中的分离性能。
3)探索该策略在其他气体分离体系中的应用。
Machine Learning-Assisted Discovery of Propane-Selective Metal−Organic Frameworks
文章作者:Ying Wang, Zhi-Jie Jiang, Dong-Rong Wang, Weigang Lu*, and Dan Li*
DOI: 10.1021/jacs.3c14610
文章链接:https://doi.org/10.1021/jacs.3c14610